Deploying artificial intelligence for dynamaic resource management_By Pradeep Reddy Varakanthanm_MBA
등록일 / 수정일
페이지 / 형식
자료평가
구매가격
- 2023.02.12 / 2023.02.12
- 3페이지 /
pdf (아크로벳 파일)
- 평가한 분이 없습니다. (구매금액의 3%지급)
- 2,000원
최대 20페이지까지 미리보기 서비스를 제공합니다.
자료평가하면 구매금액의 3%지급!


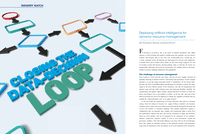
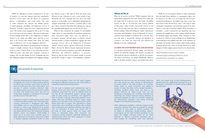
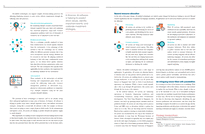
추천 연관자료
- 하고 싶은 말
- 핀란드 Aalto 대학교 MBA 과제
- 본문내용
-
INDUSTRY WATCH
By Pradeep Reddy Varakantham
For businesses everywhere, this is the epoch of artificial intelligence (AI). Major
advances in deep learning and machine learning mean that machines can now dissect,
evaluate, and interpret data in ways that were inconceivable just a decade ago. As
a result, companies across all industries are harnessing AI to become more productive,
to predict better and to market better. While we have been using computers for over
six decades to help with business decision-making, today we find that AI systems are
demonstrably delivering state-of-the-art performance for enabling business decisions,
notably in resource planning, deployment and management.
The challenge of resource management
Every business is faced with the most basic, and also the most complex, decision of
optimally allocating its limited resources, both human and non-human, to meet multiple
demands in a way that brings maximum benefit to stakeholders. On the factory floor,
this takes the form of scheduling labour and allocating raw materials to achieve the target
output in the most efficient manner. In the warehouse, this calls for arrangements that
optimise space and time while reducing errors and improving flexibility. Similarly, the
transportation and logistics sphere requires optimal allocation of vehicles to ensure the
fastest and cheapest ways to get products to market. At the last mile, sales and service
delivery personnel also need to be deployed in a similar way, regardless of whether they are
working for a brick-and-mortar store or an online business.
In the real world, this optimisation of resource allocation takes place in a dynamic
setting, where the volume of resources (i.e. supply of labour, machines, raw materials,
warehouse space, trucks, and service delivery personnel) and the demand for the goods and
services they produce is constantly changing. Such dynamic deployment requires a
configuration that can anticipate these changes and continuously adapt to them. Even
this optimisation sequence that takes into account uncertainty in supply and demand is
based on ceteris paribus, and can be disrupted by the emergence of new products,
markets, competitors, business models, as well as new environment, health and
governance mandates. Such adversarial in uences can throw off even the best-laid plan,
hence they require an immediate response to that particular incident. Such disruptions
range from the uberisation of the taxi industry to the recent outbreak of the coronavirus.
Deploying artif cial intelligence for
dynamic resource management.
Bike-sharing in s ingapore
Source: Pradeep Varakantham, “Using Big Data to make bike sharing more effcient”, Today, June 2017.
Where AI fts in
What do AI systems do better? While computers that run
optimisation programmes have been around for a while, and
are being used by businesses across the board, AI-enabled
systems are able to: (1) deal with scale, process millions
of data points within seconds, and provide answers in real
time; and (2) learn from past data to continuously improve
decision-making. Enhanced intelligence enables simultaneous
processing and deployment of tens of thousands of resource
segments under hundreds of scenariosall in real time. They
not only help to dynamically optimise the allocation of
resources, but allow for short-term and medium-term
planning to be done simultaneously.
Closing the loop between data and de Cisions
In a dynamic environment, demand, supply, and adversary
patterns are constantly changing. One moment, you think you
understand the universe and plan for it, and the next moment,
the market environment can change dramatically. Business
today is all about incident response and incident prevention.
Therefore, the second way was conceived, where the
onus was placed on users, instead of intermediaries,
to position bikes in the right locations. An incentive-
based system in which customers were offered a
reward to leave the bikes at the desired locations
meaning locations where there was likely to be
greater demand for bikescould work. The key
challenge in providing such monetary incentives was
balancing the trade-off between being attractive to
customers (leaving the bike at a location desired by
the bike-sharing company) and being fnancially
feasible for the bike-sharing company.
While data-based prediction methods helped predict
consumer demand, they were not suffcient to compel
customers to move bikes to desired locations. The
AI systema data-driven decision-making system
could fgure out the right fnancial incentives to use,
given the multiple scenarios based on the future
demand of rational consumers operating with a
fxed budget. By combining data and decisions
within one closed loop for bike sharing-systems,
performance was signifcantly improved by up to
40 percent, compared to methods using multiple
real data sets.
While the use of enterprise software to collect operational
data and build robust databases is commonplace tod
자료평가
-
아직 평가한 내용이 없습니다.
오늘 본 자료
더보기

최근 판매 자료
- [물류경영] 바릴라스파 `Barilla SpA` 문제점 및 해결방안
- 사우스웨스트 항공 원가우위, 차별화 전략
- [재무제표 분석] 홈쇼핑 산업분석 -GS홈쇼핑과 CJ홈쇼핑의 가치평가를 중심으로
- 이마트 E-마트의 공급사슬관리 SCM
- 스포츠마케팅_프로스포츠 구단(축구, 야구, 농구, 배구 등)을 1개 선정하여 해당 구단을 SWOT분석하여 다른 구단에 대한 경쟁전략을 수립하라 (3)
- [가치사슬, 경영전략] 가치사슬분석
- [마케팅] 아름다운 재단 SWOT 분석 및 개선 방안
- [광고홍보조사 설문지] 숙취해소음료의 제품과 광고에 대한 이미지
- [설문지] 기능성음료에 대한 마케팅 조사 설문지
- [국제기술이전] 국제기술이전의 개념과 효과, 특성(특징), 국제기술이전 구조와 경로
저작권 관련 사항 정보 및 게시물 내용의 진실성에 대하여 레포트샵은 보증하지 아니하며, 해당 정보 및 게시물의 저작권과 기타 법적 책임은 자료 등록자에게 있습니다. 위 정보 및 게시물 내용의 불법적 이용, 무단 전재·배포는 금지됩니다. 저작권침해, 명예훼손 등 분쟁요소 발견시 고객센터에 신고해 주시기 바랍니다.